Prediction of Ultimate Compressive Strength of CCFT Columns Using Machine Learning Algorithms
Само за регистроване кориснике
2022
Конференцијски прилог (Објављена верзија)
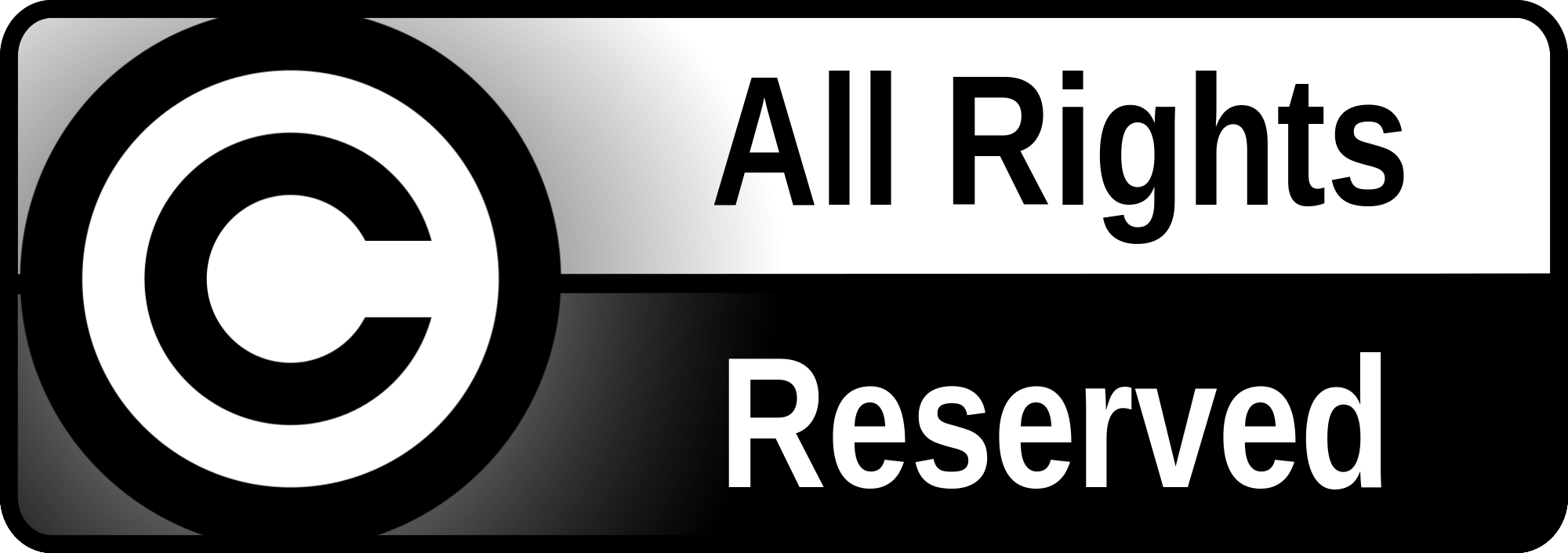
Метаподаци
Приказ свих података о документуАпстракт
The composite concrete-filled steel tube columns are structural members with numerous advantages over the traditional reinforced concrete or the pure steel members. The behavior of these columns is highly nonlinear. This paper analyses the determination of the ultimate compressive capacity of circular concrete-filled tubes according to the Eurocode 4 design code and its accuracy in comparison with the experimentally available results from the literature. In order to improve the predictions of the column’s ultimate compressive capacity, two efficient machine learning algorithms are employed separately for the stub and the slender columns. The analyzed algorithms are the Decision tree and the Random forest. The research used an experimental dataset of 508 samples: 236 tests on the stub columns and 272 tests on the slender columns. It adapted the dataset to the provision of the Eurocode 4 design code. The predictions of the column’s ultimate axial capacity obtained by two ML algorithms an...d the Eurocode 4 are compared with the experimental test results on the validation dataset. The calculated R2 error measure has shown that the predictions obtained by the tree-based algorithms are superior compared to the design formulas offered by the Eurocode 4. The decision tree algorithm achieved the best accuracy measured with the highest value of R2 error measure between the two algorithms. The two analyzed algorithms are used to perform the sensitivity analysis on the considered problem. The sensitivity analysis used the feature importance, a technique that assigns a score to each of the input features based on their influence on the prediction of the output variable. As expected, the sensitivity analysis identified the outer diameter of the columns’ cross-section as the parameter with the most significant impact on the results.