Refinement of Individual Tree Detection Results Obtained from Airborne Laser Scanning Data for a Mixed Natural Forest
Само за регистроване кориснике
2022
Аутори
Brodić, Nenad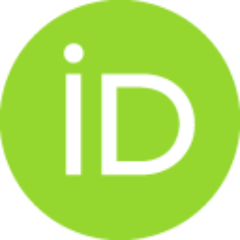
Cvijetinović, Željko
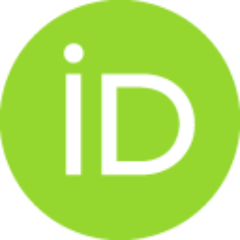
Milenković, Milutin
Kovačević, Jovan
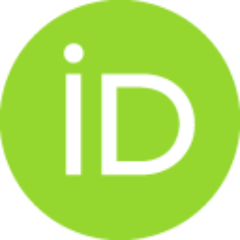
Stančić, Nikola
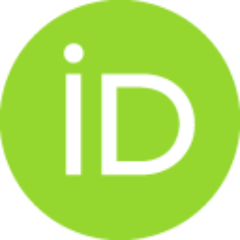
Mitrović, Momir
Mihajlović, Dragan
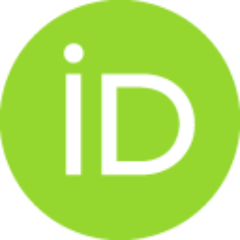
Чланак у часопису (Објављена верзија)
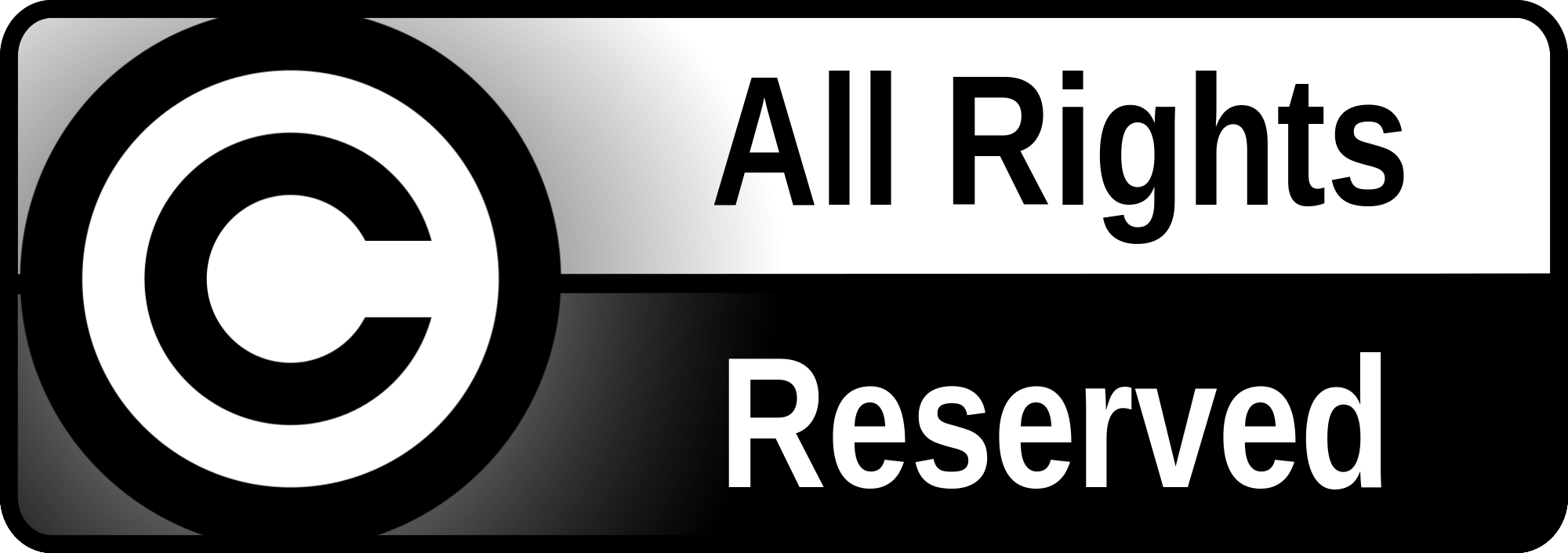
Метаподаци
Приказ свих података о документуАпстракт
Numerous semi- and fully-automatic algorithms have been developed for individual tree detection from airborne laser-scanning data, but different rates of falsely detected treetops also accompany their results. In this paper, we proposed an approach that includes a machine learning based refinement step to reduce the number of falsely detected treetops. The approach involves the local maxima filtering and segmentation of the canopy height model to extract different segment level features used for the classification of treetop candidates. The study was conducted in a mixed temperate forest, predominantly deciduous, with a complex topography and an area size of 0.6 km × 4 km. The classification model’s training was performed by five machine learning approaches: Random Forest (RF), Extreme Gradient Boosting, Artificial Neural Network, the Support Vector
Machine, and Logistic Regression. The final classification model with optimal hyperparameters was adopted based on the best-performing cl...assifier (RF). The overall accuracy (OA) and kappa coefficient (κ) obtained from the ten-fold cross validation for the training data were 90.4% and 0.808, respectively. The prediction of the test data resulted in an OA = 89.0% and a κ = 0.757. This indicates that the proposed method could be an adequate solution for the reduction of falsely detected treetops before tree crown segmentation, especially in deciduous forests.
Кључне речи:
individual tree detection / airborne laser scanning / machine learning / Random Forest / Extreme Gradient Boosting / artificial neural network / Support Vector MachineИзвор:
Remote Sensing, 2022, 14(21), 5345, 2022, 14Издавач:
- MDPI
Финансирање / пројекти:
- Министарство науке, технолошког развоја и иновација Републике Србије, институционално финансирање - 200092 (Универзитет у Београду, Грађевински факултет) (RS-MESTD-inst-2020-200092)
Институција/група
GraFarTY - JOUR AU - Brodić, Nenad AU - Cvijetinović, Željko AU - Milenković, Milutin AU - Kovačević, Jovan AU - Stančić, Nikola AU - Mitrović, Momir AU - Mihajlović, Dragan PY - 2022 UR - https://grafar.grf.bg.ac.rs/handle/123456789/2775 AB - Numerous semi- and fully-automatic algorithms have been developed for individual tree detection from airborne laser-scanning data, but different rates of falsely detected treetops also accompany their results. In this paper, we proposed an approach that includes a machine learning based refinement step to reduce the number of falsely detected treetops. The approach involves the local maxima filtering and segmentation of the canopy height model to extract different segment level features used for the classification of treetop candidates. The study was conducted in a mixed temperate forest, predominantly deciduous, with a complex topography and an area size of 0.6 km × 4 km. The classification model’s training was performed by five machine learning approaches: Random Forest (RF), Extreme Gradient Boosting, Artificial Neural Network, the Support Vector Machine, and Logistic Regression. The final classification model with optimal hyperparameters was adopted based on the best-performing classifier (RF). The overall accuracy (OA) and kappa coefficient (κ) obtained from the ten-fold cross validation for the training data were 90.4% and 0.808, respectively. The prediction of the test data resulted in an OA = 89.0% and a κ = 0.757. This indicates that the proposed method could be an adequate solution for the reduction of falsely detected treetops before tree crown segmentation, especially in deciduous forests. PB - MDPI T2 - Remote Sensing, 2022, 14(21), 5345 T1 - Refinement of Individual Tree Detection Results Obtained from Airborne Laser Scanning Data for a Mixed Natural Forest VL - 14 DO - 10.3390/rs14215345 ER -
@article{ author = "Brodić, Nenad and Cvijetinović, Željko and Milenković, Milutin and Kovačević, Jovan and Stančić, Nikola and Mitrović, Momir and Mihajlović, Dragan", year = "2022", abstract = "Numerous semi- and fully-automatic algorithms have been developed for individual tree detection from airborne laser-scanning data, but different rates of falsely detected treetops also accompany their results. In this paper, we proposed an approach that includes a machine learning based refinement step to reduce the number of falsely detected treetops. The approach involves the local maxima filtering and segmentation of the canopy height model to extract different segment level features used for the classification of treetop candidates. The study was conducted in a mixed temperate forest, predominantly deciduous, with a complex topography and an area size of 0.6 km × 4 km. The classification model’s training was performed by five machine learning approaches: Random Forest (RF), Extreme Gradient Boosting, Artificial Neural Network, the Support Vector Machine, and Logistic Regression. The final classification model with optimal hyperparameters was adopted based on the best-performing classifier (RF). The overall accuracy (OA) and kappa coefficient (κ) obtained from the ten-fold cross validation for the training data were 90.4% and 0.808, respectively. The prediction of the test data resulted in an OA = 89.0% and a κ = 0.757. This indicates that the proposed method could be an adequate solution for the reduction of falsely detected treetops before tree crown segmentation, especially in deciduous forests.", publisher = "MDPI", journal = "Remote Sensing, 2022, 14(21), 5345", title = "Refinement of Individual Tree Detection Results Obtained from Airborne Laser Scanning Data for a Mixed Natural Forest", volume = "14", doi = "10.3390/rs14215345" }
Brodić, N., Cvijetinović, Ž., Milenković, M., Kovačević, J., Stančić, N., Mitrović, M.,& Mihajlović, D.. (2022). Refinement of Individual Tree Detection Results Obtained from Airborne Laser Scanning Data for a Mixed Natural Forest. in Remote Sensing, 2022, 14(21), 5345 MDPI., 14. https://doi.org/10.3390/rs14215345
Brodić N, Cvijetinović Ž, Milenković M, Kovačević J, Stančić N, Mitrović M, Mihajlović D. Refinement of Individual Tree Detection Results Obtained from Airborne Laser Scanning Data for a Mixed Natural Forest. in Remote Sensing, 2022, 14(21), 5345. 2022;14. doi:10.3390/rs14215345 .
Brodić, Nenad, Cvijetinović, Željko, Milenković, Milutin, Kovačević, Jovan, Stančić, Nikola, Mitrović, Momir, Mihajlović, Dragan, "Refinement of Individual Tree Detection Results Obtained from Airborne Laser Scanning Data for a Mixed Natural Forest" in Remote Sensing, 2022, 14(21), 5345, 14 (2022), https://doi.org/10.3390/rs14215345 . .